AI has made significant strides in developing large language models (LLMs) that excel in complex tasks such as text generation, summarization, and conversational AI. Models like LaPM 540B and Llama-3.1 405B demonstrate advanced language processing abilities, yet their computational demands limit their applicability in real-world, resource-constrained environments. These LLMs are often cloud-based, requiring extensive GPU memory and hardware, which raises privacy concerns and prevents immediate on-device deployment. In contrast, small language models (SLMs) are being explored as an efficient and adaptable alternative, capable of performing domain-specific tasks with lower computational requirements. The primary challenge with LLMs, as addressed by SLMs,
A Deep Dive into Small Language Models: Efficient Alternatives to Large Language Models for Real-Time Processing and Specialized Tasks
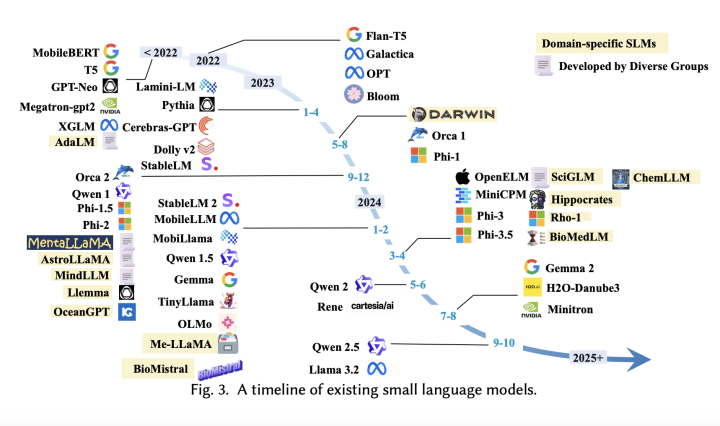
This was originally published on post